How AI and Data Science are driving finance transformation
Aug 2023
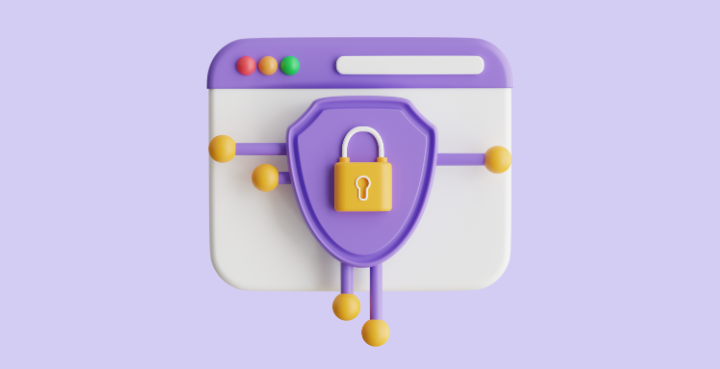
Under the hood of Xelix's Accounts Payable Control Centre
By combining rule-based algorithms and machine learning models, Xelix has become a leader in detecting anomalies within Accounts Payable data. Central to our anomaly detection expertise is advanced modelling techniques with Artificial Intelligence (AI).
This is where our Machine Learning models come into play, recognising subtle and nuanced patterns in financial data. By virtue of these advanced AI techniques, Xelix can detect more errors than purely rule-based systems, like your ERP or Excel. With a total of 60 models - each tailored to specific error-detection functions - our platform's capability is unmatched.
Data Science at Xelix
At Xelix, we believe that the power of data science lies not just in complex algorithms and fancy models, but in their practical application to solve real-world problems. Our in-house Data Science team, tech stack, and range of AI models are all guided by this philosophy.
From error detection to reconciliation, our mission is to streamline financial data management and make it as accurate and efficient as possible. Because we know that finance teams just don’t have the time to be burdened with inaccurate data and inefficient solutions.
Let's dive into some of the specific areas where Xelix excels in data science.
Duplicate invoice detection
Xelix's Transactions module is designed to identify errors and duplicate invoices in ERP systems. This is a big deal for companies, especially large ones with numerous suppliers and a high volume of invoices. Xelix uses advanced Machine Learning (ML) techniques, specifically Gradient Boosting*, to sift through massive amounts of data.
*Gradient Boosting is a machine learning technique used in regression and classification tasks, among others. The Gradient Boosting technique is an ensemble method, which means that multiple models are trained on the data, with each successive model improving upon the previous one. Finally, the knowledge and experience of all these models are combined to create a new, more powerful model.
As duplicate invoices can arise from a number of different processes, Xelix use several different models in our duplicate detection process, with each model fine-tuned to identify a different variety of duplicate.
For example, one model is designed to detect duplicates caused by typographical errors, i.e., when entering invoice numbers, while another detects duplicates where the same invoice has been posted to multiple vendors. Xelix combines over 27 specialised models to accurately detect these duplicates.
We can also develop customer-specific models to further enhance their AP performance and accuracy. There are 18 such models in operation, each fine-tuned to the customer’s unique data requirements. Combined, these models help our customers find errors in their ERP systems, identify opportunities for recovering lost capital, and avoid making these payments in the future.
Automated supplier statement reconciliation
Our Statements module automates the reconciliation process and checks statements against our customers' ERP systems. It can be a big headache for Accounts Payable teams to manually process statements and ensure everything matches up with their ledger.
Xelix’s automated supplier statement reconciliation module aims to make this process touchless and efficient. We use Computer Vision Convolutional Neural Networks (CNN) to process both PDF and spreadsheet statements. Computer Vision is a field in AI that allows computers to interpret visual information, like pictures or documents, and extract data from them, while Convolutional Neural Networks are deep learning techniques used for image analysis.
Our automated supplier statement reconciliation module uses these techniques to identify where important information is located on a document, such as columns indicating dates or sales tax.
Plus, Xelix can highlight invoices on the statement that have already been processed, that are missing from the system, or that still need to be processed.
Continuous improvement
Data is more than just numbers for Xelix - it's the driving force behind our model refinement and improvement. In 2023, Xelix customers have provided us with an average of 3.9 million invoices every month. That's a lot of data. We use this data to enhance our duplicate invoice detection and automated reconciliation capabilities. But it doesn't end there.
Customers use feedback buttons (Yes / No / Ignore) within the Xelix platform, which helps the Xelix AI to continuously improve both the user experience and the accuracy of the models themselves. This ultimately drives fewer false positives (inaccurate results), which other tools deliver in abundance.
Data privacy and security
At Xelix, we truly value our customers' data privacy and security. The data is stored securely and carefully maintained by our talented team of engineers. We’re all about using data to fuel progress and ensure our customers get the best possible service.
Xelix in action
Xelix is taking financial data management to new heights with our advanced AI and data science techniques. We’re committed to making things easier, more accurate, and more efficient by delivering practical solutions to real-world problems.
And with Xelix’s customer-centric approach, we’re constantly improving and redefining what's possible in the world of financial technology.
If you are interested to see how all this looks in action, book your Xelix demo today.
Our Data Science team
We can't forget about the fantastic team behind Xelix's data science magic. Led by Paul Solomon, Head of Data Science. Xelix’s Data Science team is composed of diverse individuals with impressive backgrounds.
Paul has extensive commercial experience leading data science teams at Aviva and IPG Mediabrands. He's an expert in applying Machine Learning and AI to real-world business problems.
Zoran Medić, who gained expertise in natural language processing during his Ph.D. in Computing is focused on training deep learning models to enhance citation recommendations in scientific articles.
James Farr, who completed a Ph.D. in Astrophysics and used machine learning to classify astrophysical bodies, is a pro at developing new data analysis techniques.
Claire Smid, who earned her Ph.D. in Cognitive Neuroscience, focused on reinforcement learning and decision-making in the brain. Claire has published scientific papers and led several computer science workshops.
Together, this team constantly pushes the boundaries of innovation to ensure that Xelix stays at the forefront of data science.
Content that may catch your eye
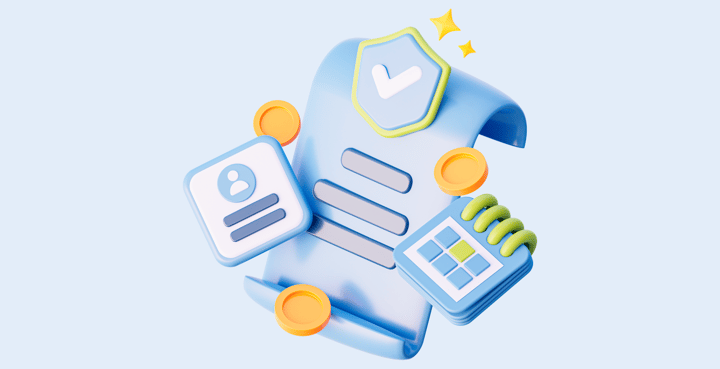
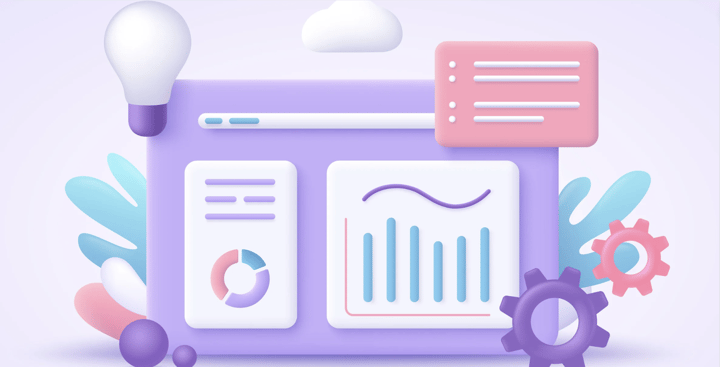
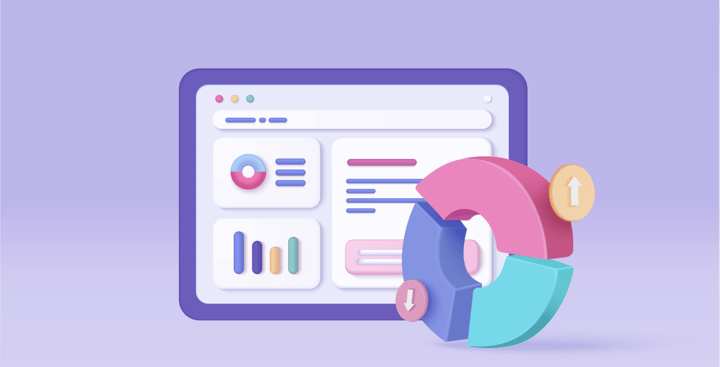