Using AI to build world-leading duplicate invoice prevention software
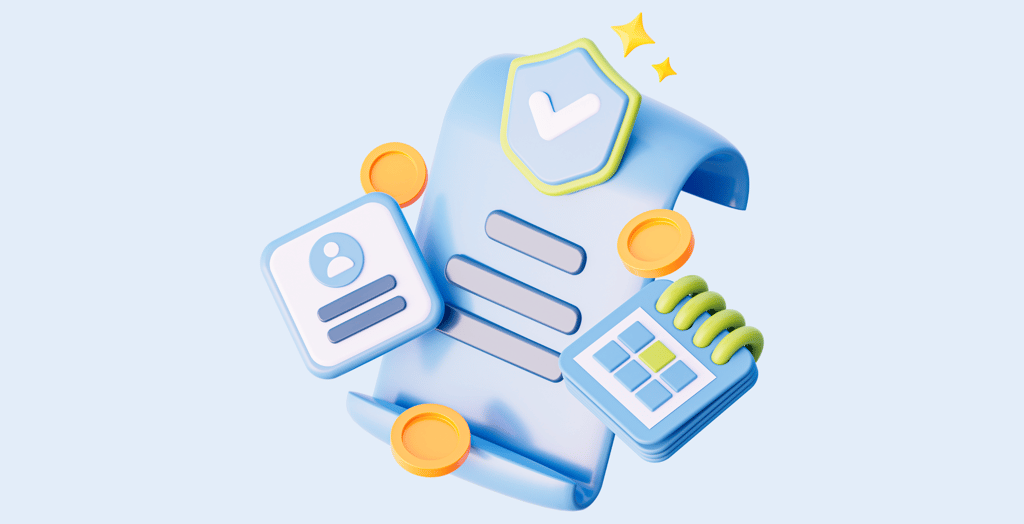
Despite the marketing headlines, most – if not all – players in the duplicate invoice prevention market use rules-based tools to detect errors. The complexity and variety of data from different organisations around the world, however, mean this “one size fits all” approach is simply not efficient. High numbers of inaccurate results (false positives) are surfaced, which creates huge amounts of additional work for Finance teams.
This blogpost explains how Xelix solved this problem by creating the next generation of duplicate invoice prevention software using AI.
Rules Based Detection – The Status Quo
When uncovering potential invoice duplication, most rules-based systems work by fixing certain invoice fields and allowing one to vary within a set of defined parameters. An example might be: find all invoices where the supplier, the invoice date and the invoice amount match but the invoice number is slightly different. Typically, a fuzzy matching score or a string distance algorithm is used, and a threshold set to define how similar the two invoice numbers need to be for the pair to get flagged as potential duplication.
And certainly, looking for a slight variation in the invoice number is a good place to start. In most circumstances, an exact match on the invoice number is the only check ERPs do to prevent the same invoice from being posted twice. In fact, at Xelix, the data shows that a large proportion of duplicate postings is due to one item having an error somewhere within the invoice number, which is why it bypassed the basic ERP controls in the first place. We see whole host of errors here, from ones which are seemingly random to the classic keying in/scanning character substitutions (O->0, I->l, B->8 etc).
But as anyone who works with a rules-based system will tell you, the vast majority of their time is spent sifting through all the false positives versus actually removing true duplicated items.
How is Xelix Different?
At Xelix, we have adopted a far more sophisticated approach. First, we “throw a wide net” by using a complex rules-based system to quickly identify a large pool of risky transactions. Second, we use our AI engine to forensically remove the false positives from the pool. This is where our technology approach comes to life. Specifically, we utilise five different tree boosting Machine Learning models, interrogating over 300 unique data points per invoice.
After surfacing potential duplicates, we ask users to action items in the platform to confirm if the models were correct and this completes the feedback loop. The responses are then added to the training datasets allowing our models, which use supervised learning, to become more accurate over time as they are continuously re-trained.
Another key difference is that Xelix reviews all invoice data every day. This means that as you process more invoices from a supplier, a potential duplicate invoice from yesterday may no longer look like a duplicate today, because a clearer invoicing pattern has emerged. Think about reoccurring monthly rent invoices for example, where the second monthly invoice looked like a duplication of the first but after month six, it’s clear that the similar postings are intentional.
How does our AI help to detect duplicate invoices?
1. Data preparation and rules-based detection
Given Xelix assesses all your invoice data every day, this represents a complex engineering challenge. Imagine your business processes 1m invoices every year. After year one, there will be 500 billion combinations in which two invoices could be paired for review. If you look at 1,000 of those every second, it would still take nearly 16 years to check them all.
Using our advanced mechanism for prepping the data to produce pairs of invoices for review, Xelix makes use of a number of algorithms to compare the two invoices in question. One of these is a highly customised version of the Damerau–Levenshtein string distance algorithm for matching the invoice numbers. Large datasets are quickly whittled down to leave only high-risk transactions.
2. The AI: removal of false positives
Step 2 is to pass the results to five machine learning models and one proprietary Xelix algorithm for further review. This part of the process involves converting each invoice pair into over 300 data points that contain information about how the two invoices compare and, as importantly, how the invoices relate to other invoices from the supplier and the wider dataset. Within these data points lies all the required information for the models to make an accurate prediction.
To appreciate how powerful this is, imagine if the potential duplicate invoice belongs to a supplier with 100k other invoices. Our design and elegant engineering means the invoice in question is assessed against its potential match in the context of all 100k invoices at the same time and in a fraction of a second by the ML models – something that would clearly be impossible for a human to do in a timely and accurate way.
3. Risk classification
After the false positives are removed, the last step of the process is to risk rate each of the pairs into a High / Medium / Low category to help our users to prioritise which to investigate first. Given the complexity of the various outputs from earlier parts of the process, we use a sixth ML model to make this decision.
The end result is the world’s most sophisticated duplicate detection model – surfacing errors missed by other tools and doing so with a radically improved accuracy rate.